Predictive Machine: The Harvard Business Review's new book, Simple Economics of Artificial Intelligence, presents an interesting argument. The three economists from the Rotman School of Management in Toronto, through current machine intelligence and automated propaganda, have identified the surprisingly simple economy behind the rapid rise of advanced analytics and machine learning technologies that we now typically classify as "AI." Claim. Intelligent systems generate lower forecasting costs, and there is a power of change.
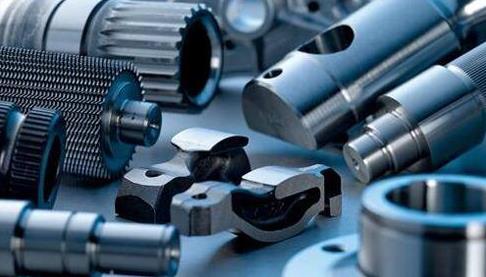
Manufacturing is no stranger to the brilliance of automation, but predictive technology brings higher value. Intelligent systems reduce operational uncertainty by collecting and executing calculations of relevant data. This increases efficiency and productivity and creates opportunities for new business strategies and cost reductions. As a result, the global manufacturing analytics market is expected to grow at a CAGR of 22% in 2024 due to the growing Industrial Internet of Things (IIoT), the widespread adoption of advanced data management strategies, and the ongoing demand for process optimization. A 2018 digital supply chain implementation survey found that 50% of manufacturers see agility and innovation as their primary focus, and see real-time product visibility as the primary driver of investment (40% driven by rapid innovation demand). 33% of the drivers reduce costs by improving planning).
While some forms of traditional and/or cloud-based analytics enable manufacturing companies to better master and manage their existing processes, predictive technologies reliably predict future performance and enable organizations to preempt these forecasts using real-time data. For example, the recent TDWI Navigator report highlights one of the most compelling advantages of manufacturing forecasting - the ability to monitor machines and use past data to predict or prevent future failures. The popular IIoT predictive maintenance use case involves sensors tracking operating parameters and machine conditions in high value equipment. This provides the ability to predict possible part failures and can be used to improve production uptime:
That "embedded" bit is where things get complicated. In order to automate operations when needed, the prediction system must also respond in an environment where data is generated, usually in real time. Standard cloud management, batch-oriented analysis cannot achieve this. The time and expense of collecting, transmitting, processing, routing and responding to any information collected from the vast amounts of data generated in the IIoT sensor network negates any value that can be captured if only relying on the cloud - it has no benefit in the engine card After two hours of inactivity, let the “AI†alert the operator to an impending device failure. To facilitate real-time decision making, action or insight, the architecture must also support flow analysis (calculation of data execution as it flows through the system) and traditional cloud analysis, combining real-time and historical analysis techniques. Models that explore the past and use this information to optimize functionality can be cloud-driven, but the predictive edge analysis framework that consumes sensor or device data streams when ingesting provides the necessary real-time operational insight when events occur.
Increasingly compact rules engines and pattern libraries facilitate this type of intelligence directly on devices in a manufacturing environment, allowing systems to ask complex problems and return immediate results and/or take immediate action.
Integrating computational intelligence into the "edge" of operations (the network meets the real world) extends predictive capabilities to tangible actions where data-based insights are used as needed times and places. Such systems predict and prevent failures and ensure optimal value. Whether it is applied to the supply chain, asset maintenance and management, product development automation or planning - this is a mechanism to reduce the cost of forecasting and "artificial intelligence" to prove its value.
Economics is simple, but implementation is not. You can't simply order an "AI" system and automatically become a "smart" factory with predictive capabilities. All of these techniques are based on rules. Defining these rules requires manpower. In order for manufacturers to benefit from predictive technology, they must be able to clearly identify and specify what they need to predict and then build a customized solution from there. When you specify these goals and objectives, your clear process is different for each organization, but one thing is certain. Future business requires not only the most advanced engines, pumps, processes and systems to succeed. They will need the most advanced assets and predictive intelligence to maintain, service and optimize them.